
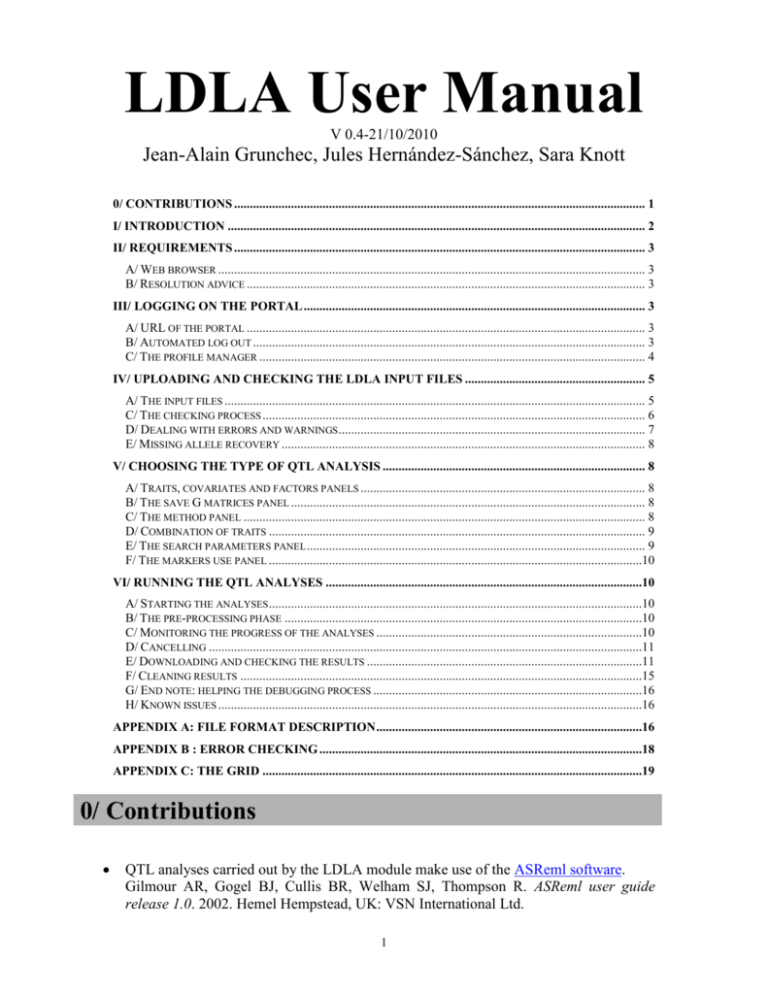
However, growth of this level in any industry, whilst exciting and positive in many ways, also brings with it its own complications. This rise in aquaculture production isn’t much of a surprise when you consider the emphasis on the health-giving properties of fish (particularly Omega-3 from oily fish) and the subsequent encouragement for people to eat more oily fish and it’s certainly good news for the aquaculture producers across the world. It is a rapidly growing division of farming across the world reports from the UN’s FAO suggest that it has been growing more rapidly than any other area of animal food production.Ī report published in September 2009 suggested that farm-reared fish accounted for 50% of world fish consumption. Below are some example gains the ASReml team were able to quantifyĪquaculture is the name given to the farming of salt water and freshwater fish and marine animals. These gains depend upon a variety of factors including: microprocessor, machine power, dataset size and type of analysis run, among others, and they will vary between users. ASReml-SA 4.2 - processing speed gains ASReml-SA 4.2 delivers impressive gains in processing speed.
ASREML PLUS PLUS
Plus with parallel processing and the ability to allocate memory to certain tasks, we've also made it much faster: check out the comparison table below to see speed gains we've achieved for a selection of analyses. New release for 2021 Our latest release, ASReml-SA 4.2, has three times as much available memory as 4.1 and can therefore handle much larger analyses. Linear mixed effects models provide a rich and flexible tool for the analysis of many data sets commonly arising in animal, plant and aqua breeding, agriculture, environmental sciences and medical sciences.
ASREML PLUS SOFTWARE
These steps are somewhat similar to those for the nlme.VC.Harness the power of REML ASReml-SA is powerful statistical software specially designed for mixed models using Residual Maximum Likelihood (REML) to estimate the parameters. It should be noted, however, that the estimates for mod4 do need further formatting in order to obtain the here presented results.
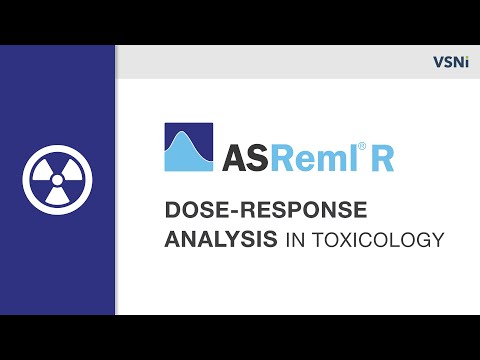
This was already achieved via the ods output covparms=modsasVC lines in the PROC MIXED statements in the previous section. Thanks to the ODS (Output Delivery System) in SAS, there are no extra steps required to extract the variance component estimates. We did not use it here, however, since it only seems to provide the sigma estimate and not the varStruct estimates (see github issue).
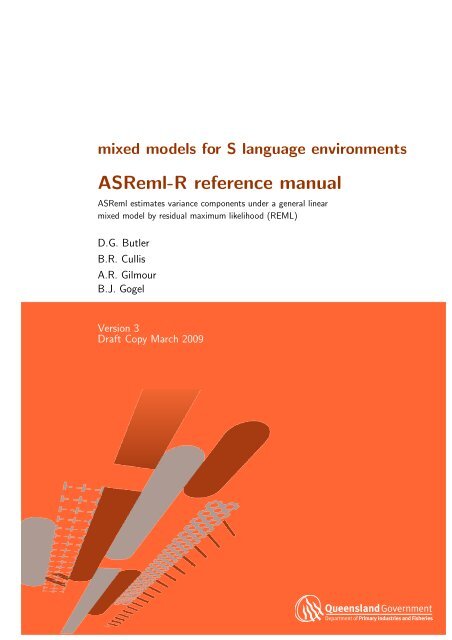
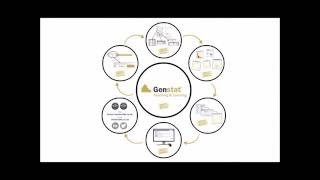
We want to point out that the tidy(effects="ran_pars") function of the broom.mixed package (which we used it in this chapter to obtain the variance component estimates from glmmTMB() models) actually works on lme() objects as well (but it does not on gls() and nlme()). % coef( unconstrained = FALSE, allCoef = TRUE) %>% enframe( name = "grp", value = "varStruct") %>% separate(grp, sep = "", into = c( "grpA", "grpB")) %>% mutate( sigma = me $sigma) %>% mutate( StandardError = sigma * varStruct) %>% mutate( Variance = StandardError ^ 2) , y =, by = "grpB") %>% mutate( sigma = me $sigma) %>% mutate( StandardError = sigma * varStructA * varStructB) %>% mutate( Variance = StandardError ^ 2) % coef( unconstrained = FALSE, allCoef = TRUE) %>% enframe( name = "grpA", value = "varStructA") % coef( unconstrained = FALSE, allCoef = TRUE) %>% enframe( name = "grpB", value = "varStructB") % rename( grpA = Var1, grpB = Var2) %>% left_join( x =. We achieve this by first creating a unit column in the data with different entries for each data point: By taking both of these actions, we are essentially mimicing the error (variance) as a random effect (variance). Thus, when doing so, we need to make sure to also add a random term to the model with the desired variance structure. We can, however, “ fix the residual variance to be 0 (actually a small non-zero value)” and therefore “ force variance into the random effects” (glmmTMB RefMan) via adding the dispformula = ~ 0 argument. Accordingly, it cannot be used to allow for heterogeneous error variances in this package. Following this trail, the glm documentation description for the weights= argument is “an optional vector of ‘prior weights’ to be used in the fitting process. In glmmTMB, the RefMan only states “weights, as in glm. In nlme, it requires “an optional varFunc object or one-sided formula describing the within-group heteroscedasticity structure” (nlme RefMan) and we make use of this in the chapter at hand. However, to our understanding, they have different functions: Just like in nlme, there is a weights= argument in glmmTMB(). In glmmTMB() it is -to our knowledge- not possible to adjust the variance structure of the error.
